Building a Multi-modal Production RAG
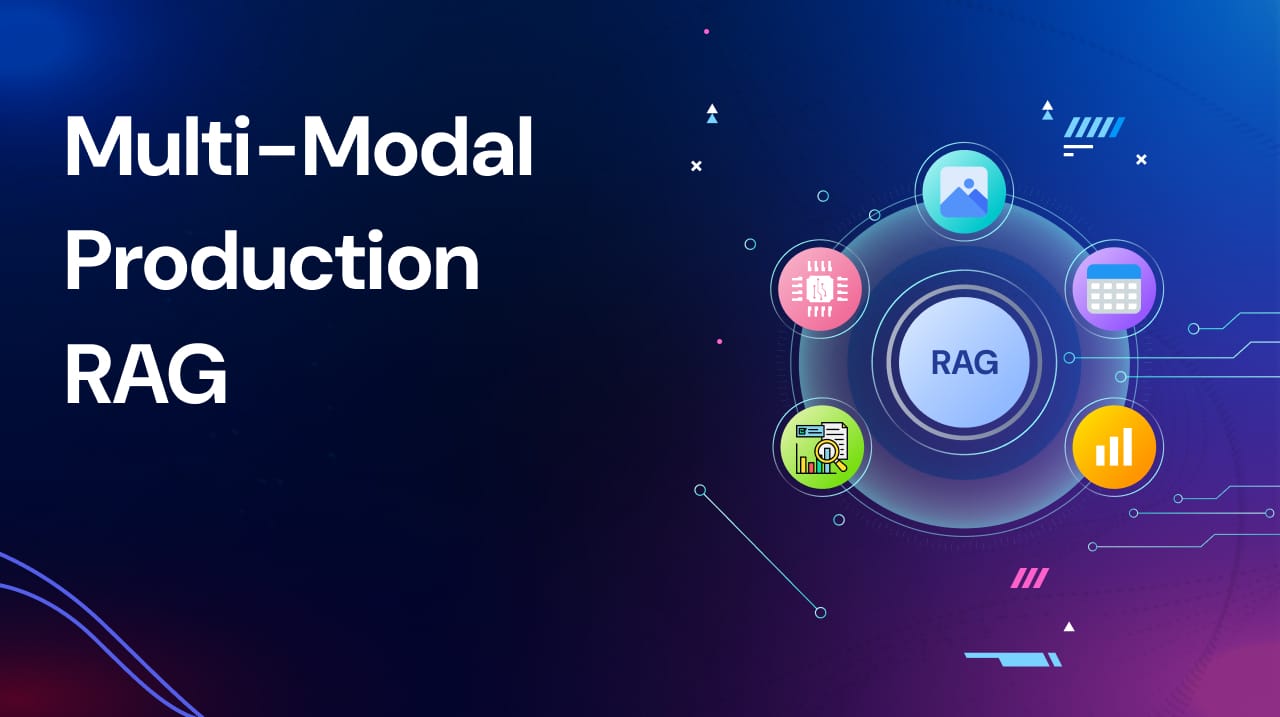
Retrieval-Augmented Generation (RAG) has rapidly become one of the most popular Gen AI systems over the past year. Initially, RAG (Retrieval-Augmented Generation) gained traction for its ability to index and retrieve unstructured data, enabling capabilities such as summarization and Q&A over textual documents. This laid the groundwork for creating simple agents that can effectively retrieve information and provide answers using the power of Large Language Models (LLMs).
Why Multi-modal RAG?
However, we are now entering an era of advanced Agentic AI, which demands sophisticated retrieval capacities that encompass not just text but also images, charts, tables, and other contextual information. Real-world documents are inherently complex; they contain a mix of text, visuals, and structured data, including scanned documents and infographics.
In such scenarios, traditional RAG systems fall short. They lack the advanced capabilities needed to process and synthesize multi-modal data effectively. To meet the challenges posed by these complex documents, developers must leverage multi-modal RAG systems that integrate and analyze diverse data formats, enabling richer insights and more accurate outputs
Real-world Scenarios for multi-modal RAG
Scenario 1: Multi-modal RAG for Analyzing Market Research Reports
Market research reports typically include a rich combination of text, images, charts, and tables, often leveraging visualizations to capture complex insights.
For instance, a consulting firm managing thousands of research reports, can significantly benefit from a multi-modal RAG system that seamlessly retrieves and integrates these diverse elements.
This AI-driven system enables consultants to extract precise insights, generate concise summaries, and answer specific questions derived from multi-modal data.
Scenario 2: RAG for Analyzing Financial Presentations
Financial presentations, including investor documents, equity research reports, etc. often feature textual data with extensive structured tables and financial charts to convey critical metrics.
In a financial services firm, analysts routinely navigate thousands of such documents for tasks like financial spreading, covenant testing, risk assessment, due diligence, and portfolio analysis.
A multi-modal RAG system empowers analysts to extract accurate data and answer specific queries related to reports, even automating tasks.
Scenario 3: Multi-modal RAG over Product Manuals
Product manuals usually consist of detailed text instructions, technical specifications, images, and diagrams.
For companies that produce technical products or machinery and require post-sales support, a multi-modal RAG system can significantly enhance user experience.
By linking textual instructions to related visuals, manufacturers empower customer support teams and end-users to quickly access essential information. This enhances onboarding and troubleshooting while reducing support ticket volumes, allowing for more effective self-service.
Multi-modal RAG on SimplAI Platform
The SimplAI platform has launched features for multi-modal RAG systems, empowering developers and product teams to build RAG pipelines over various document types, including corporate decks, research reports, product manuals, etc.
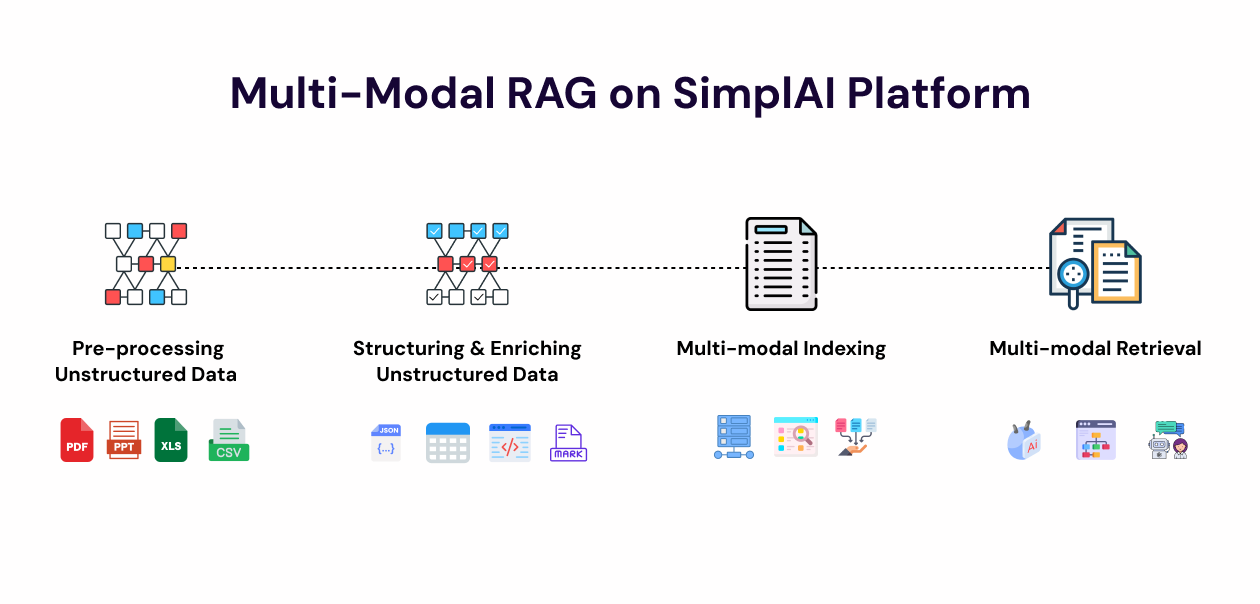
1. Pre-processing Unstructured Data
Efficient RAG systems depend on robust pre-processing. The platform effectively handles diverse unstructured documents by extracting image context and transforming tables and charts into structured formats (e.g., CSV, JSON). Various text formatting options, such as Markdown and semantic text processing, are available, ensuring minimal noise for optimal data quality.
2. Structuring & Enriching Unstructured Data
Text, images, and tables are separated into distinct sections for enhanced retrieval. Each section is enriched with sub-summaries and metadata, including page, section, and document-specific information. Timestamps, captions, and labels provide additional context, facilitating downstream retrieval and generation tasks.
3. Multi-modal Indexing
Structured data is utilized to create vector indices for different modalities, embedding text, images, and tables. Filtering options are integrated to refine search results based on user queries, improving the accuracy of retrieval.
4. Multi-modal Retrieval
Queries are expanded across modalities, leveraging learned cross-modal relationships. For instance, a text-based query regarding financial trends can retrieve both textual analysis and corresponding graphs. Post-processing logic ensures coherent retrieval fusion, generating comprehensive and relevant responses tailored to user needs.
Avoiding Hallucination
A prevalent challenge in RAG systems is hallucination, where the model generates information that lacks grounding in the retrieved data. Multi-modal RAG can help mitigate this issue by anchoring the generation process across multiple modalities.
Incorporating citations along with page screenshots enhances accuracy. For example, when analyzing financial reports, the model can cross-check its textual generation against retrieved charts or tables. This ensures that the generated content accurately reflects the underlying data, significantly reducing the risk of inaccuracies and enhancing the reliability of insights produced.
Getting Started
Unlock the power of agentic RAG with multi-modal capabilities today!
With SimplAI, you can revolutionize how your organization processes unstructured data, gaining richer insights and enhancing decision-making.
Don’t miss out on the opportunity to stay ahead in the rapidly evolving landscape of AI.
Schedule a personalized demo or consultation with our experts now, and let’s explore how we can tailor our solutions to meet your specific needs and drive your success!
Reach out to us at [email protected] if you’d like to learn more.