Ensuring Data Accuracy in AI-Driven Financial Models
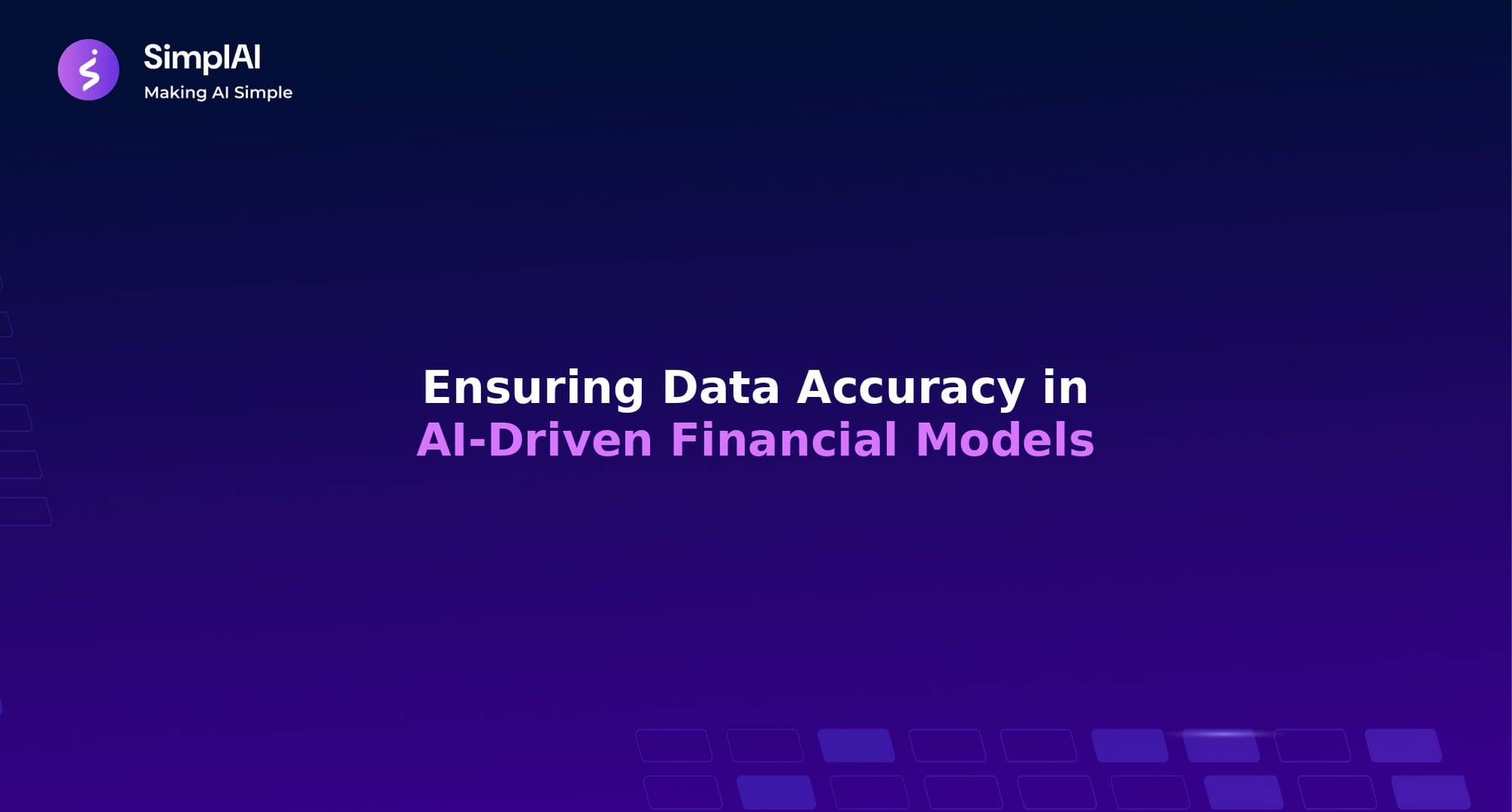
In the world of finance, where data integrity is paramount, ensuring accurate and reliable information is critical for making sound financial decisions. Artificial Intelligence (AI) is revolutionizing financial modeling, offering sophisticated approaches to prediction, analysis, and risk management. However, its true effectiveness hinges on the accuracy of the data it utilizes. As businesses strive to harness the power of AI financial modeling, addressing the challenges of data accuracy in AI has never been more crucial. This blog explores how to achieve data accuracy, the implications of poor data quality, and how SimplAI can offer solutions to help you navigate this landscape.
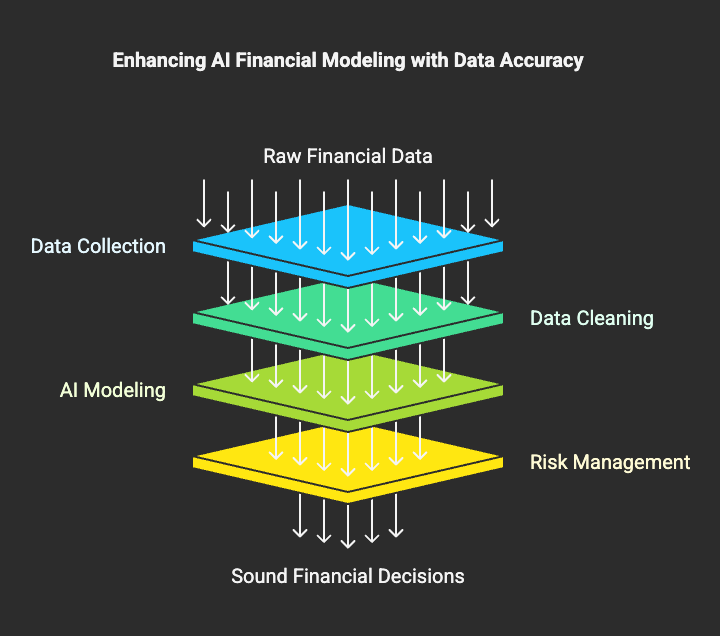
The Importance of Data Integrity
At the core of every robust financial model lies accurate and reliable data. Financial institutions increasingly rely on machine learning in finance and AI technologies to process vast amounts of data, make predictions, and automate tasks. However, as research shows, poor data quality can cost companies an average of $12.9 million each year due to misinformed decisions and compliance issues (source: Acceldata).
Successful AI-driven financial models are contingent on high-quality data, and that's where financial data integrity becomes crucial. Integration, consistency, and availability of accurate data across different financial systems must be prioritized to produce forecasts that align with reality.
Challenges in Ensuring Data Accuracy
In the realm of AI financial modeling, several challenges can compromise data accuracy:
1. Inconsistent Data Sources
Financial data often emanates from various sources, such as ERP systems, market indicators, and historical financial statements. Inconsistencies may arise if these sources are not harmonized, leading to discrepancies in forecasts.
2. Human Error
Manual data entry and processing can introduce errors that skew model outputs. It's crucial to automate data collection and validation to reduce human error and ensure data is updated in real-time.
3. Outdated Information
Relying on historical data without considering real-time market dynamics can result in inaccurate models. AI technologies must continuously refresh data to reflect current market conditions effectively.
4. Data Privacy and Compliance
In highly regulated industries, adhering to compliance standards while maintaining data integrity can be challenging. Organizations must navigate the tightrope of leveraging data analytics while protecting sensitive financial information.
AI Solutions for Enhancing Data Accuracy
To mitigate these challenges, organizations can adopt effective strategies that leverage AI capabilities:
1. Data Automation and Cleansing
AI technologies can streamline data management processes, from validating input against trusted sources to identifying and correcting discrepancies in real-time. Automated data cleansing ensures datasets are free from duplicates and inaccuracies, facilitating reliable inputs for financial modeling.
2. Predictive Analytics
By utilizing machine learning algorithms, companies can gain deeper insights into market trends and customer behavior. Not only does this help identify potential anomalies, but it also enhances the ability to forecast revenue and optimize budgeting, ultimately improving credit management automation.
3. Real-Time Monitoring
AI-driven financial platforms can provide live updates, allowing organizations to stay agile and responsive to market fluctuations. This immediacy is essential for effective risk management and informed decision-making.
4. Data Governance Frameworks
Implementing robust data governance practices can foster accountability across the organization. Establishing clear policies for data management ensures adherence to regulatory standards and enhances data quality (source: Acceldata).
Conclusion
In an age where AI is transforming financial modeling, ensuring data accuracy remains a non-negotiable cornerstone for success. Organizations that harness the benefits of machine learning, predictive analytics, and robust data governance frameworks will find themselves better equipped to navigate the complexities of financial forecasting and decision-making.
How does your organization plan to address data accuracy in AI-driven financial models? Share your thoughts in the comments below or reach out to SimplAI to learn how our solutions can help you enhance your financial data integrity.