Evaluating AI Models for Continuous Improvement: Best Practices and Techniques
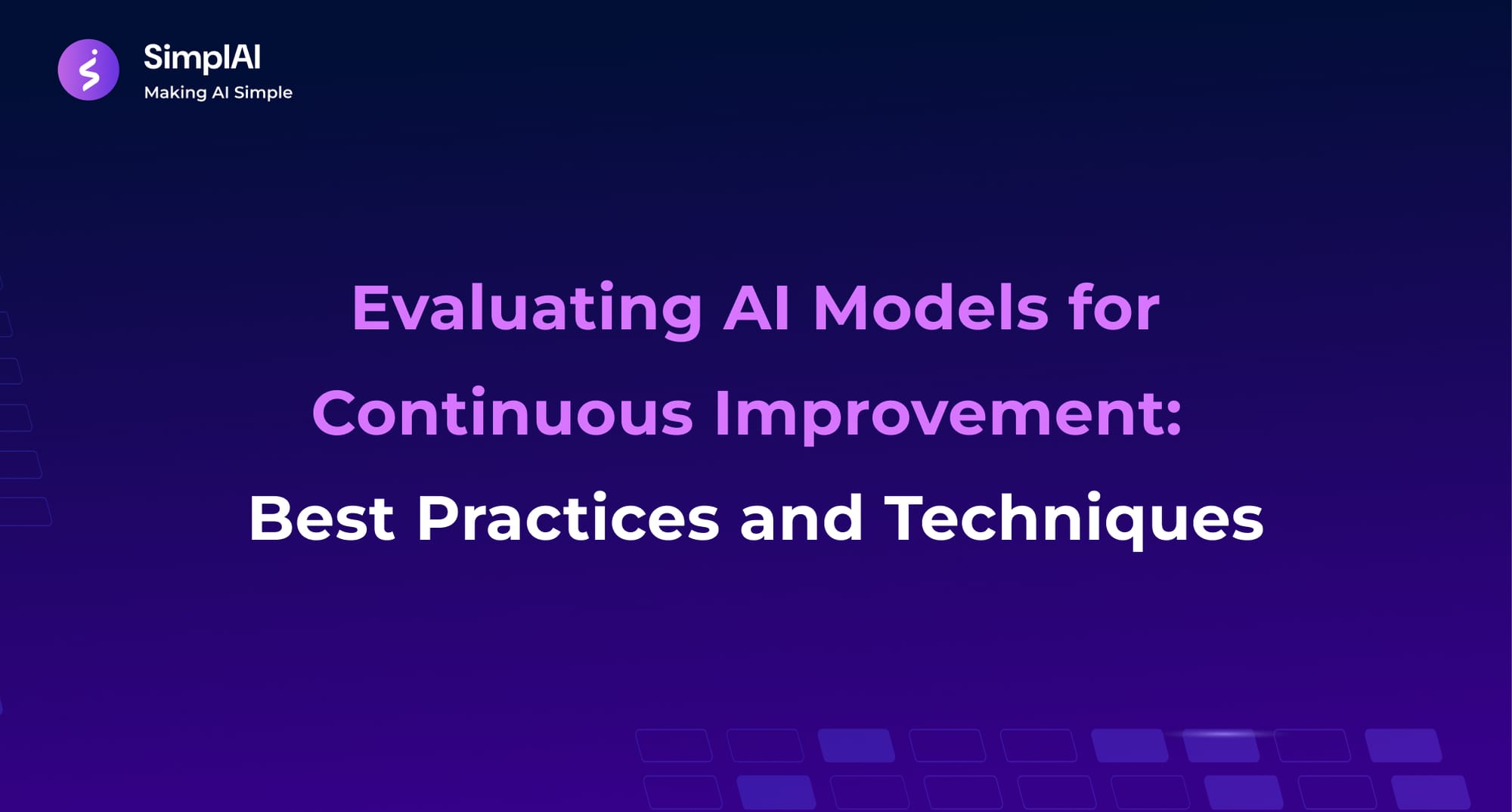
In the ever-evolving landscape of artificial intelligence (AI), ensuring the continual improvement of AI models is crucial for businesses wanting to harness their full potential. Evaluating AI models is not merely about checking for performance metrics; it's about creating a robust system that allows for ongoing enhancements, essential for tackling real-world challenges. This blog post will delve into the most effective AI model evaluation techniques, methods for continuous improvement, and how SimplAI provides solutions for optimizing AI algorithms for efficiency.
Why Evaluating AI Models is Important
As AI becomes more integrated into various sectors—ranging from healthcare to finance—effective evaluation is paramount to avoid pitfalls associated with inaccurate predictions. Poorly evaluated models can lead to costly errors, security vulnerabilities, and a loss of user trust. Understanding the nuances of AI performance metrics, such as accuracy, precision, recall, and F1 scores, enables organizations to refine their models and become more reliable in real-world applications.
Key Pain Points
- Costly Errors: In industries like finance and healthcare, inaccurate AI models can lead to disastrous financial losses or jeopardize patient lives.
- Compliance and Security Risks: Regulatory bodies impose strict guidelines on data handling and model performance. Failing to meet these can result in legal repercussions.
- Stagnation in Model Improvement: Many models become static post-deployment, leading to outdated performance and adaptability issues.
Essential AI Model Evaluation Techniques
1. Understanding Performance Metrics
Evaluating AI models requires a comprehensive understanding of performance metrics. Key metrics include:
- Accuracy: Proportion of correct predictions.
- Precision: The reliability of positive predictions.
- Recall: Ability to find relevant instances.
- F1 Score: Balances precision and recall.
- AUC-ROC: Evaluates the trade-off between false positives and true positives.
These metrics help teams determine how well a model is performing against both industry standards and specific organizational goals.
2. Data Splitting Strategies
A significant part of a reliable evaluation involves how data is segmented. Techniques such as:
- Train-Test Split: Divides data into training and test sets, providing a quick measure of model performance.
- Cross-Validation: Offers a comprehensive assessment by rotating through multiple subsets of a dataset to test a model's robustness.
Both methods ensure that models generalize well to unseen data and avoid common pitfalls such as overfitting and underfitting.
3. Continuous Monitoring and Improvement
Once deployed, AI models should be continuously monitored for performance drift. Regular evaluations allow organizations to maintain model efficiency and ensure compliance with evolving data regulations. This includes:
- A/B Testing: Comparing different models or versions to determine which performs better.
- Feedback Loops: Collecting user or system feedback to fine-tune model responses.
Implementing such adaptive strategies fosters continuous improvement in AI model performance.
Leveraging SimplAI's Solutions
At SimplAI, we recognize the value of informed decision-making in enhancing AI applications. Our platform provides tools that facilitate swift evaluations, allowing teams to build complex, high-accuracy agentic AI applications quickly and effectively.
By utilizing our solutions:
- Streamlined Evaluations: Automated tools help identify inefficiencies in performance with minimal manual intervention.
- Real-time Monitoring: SimplAI enables ongoing performance tracking, ensuring your models adapt in line with real-world conditions.
Conclusion
In conclusion, evaluating AI models is crucial for achieving continuous improvement in both performance and user satisfaction. By implementing best practices in AI model evaluation and utilizing advanced techniques, organizations can foster an environment of ongoing enhancement, ensuring that their models remain relevant and effective.
How has your organization approached AI model evaluations, and what challenges have you encountered along the way? Join the conversation by sharing your experiences below!
For those interested in maximizing the potential of their AI transformations, explore how SimplAI can simplify your AI model evaluation process. Visit us to learn more about our innovative solutions!