Detecting Insurance Fraud Using Machine Learning
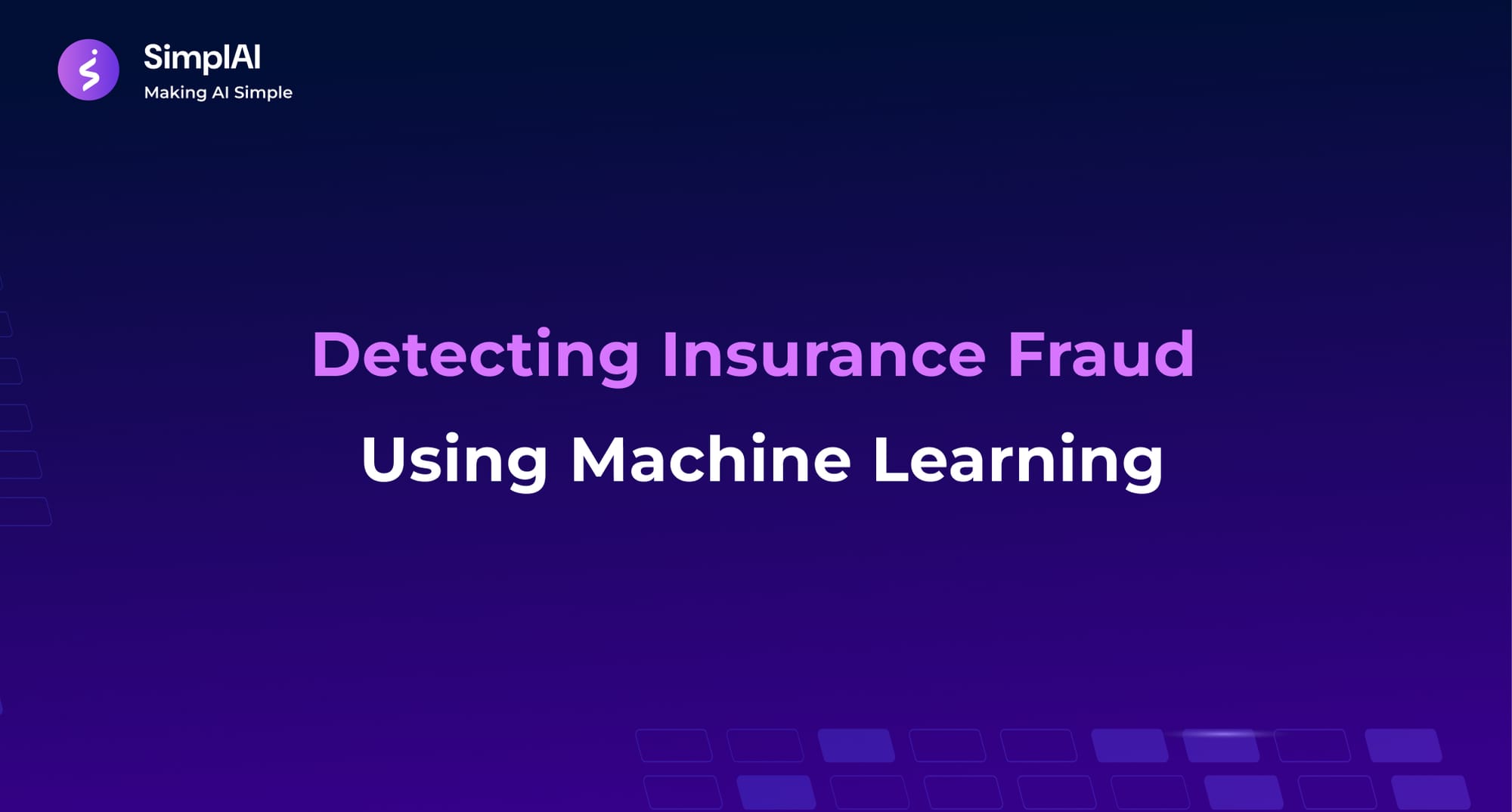
Insurance fraud is a multifaceted problem that’s costing providers billions each year, with losses exceeding $40 billion annually in the United States alone. In an age where digital transformation is reshaping every sector, the insurance industry finds itself at a crucial juncture. How can it leverage technology, especially machine learning, to combat fraud effectively? This blog explores the transformative capabilities of AI insurance fraud detection and how it can revolutionize risk management in the insurance sector.
Understanding the Landscape of Insurance Fraud
Insurance fraud encompasses a range of deceptive practices aimed at securing undeserved benefits or payouts from insurance organizations. Common methods include:
- Hard Fraud: Deliberately faking injuries or incidents to claim compensation.
- Soft Fraud: Exaggerating legitimate claims to receive higher settlements.
The complexity and evolving tactics of fraudsters make traditional detection methods, which often rely on specified heuristics and manual intervention, insufficient. Therefore, there is an urgent need to adopt a more sophisticated approach using machine learning for fraud prevention.
The Limitations of Traditional Fraud Detection
The traditional approach involves developing rules based on historical fraud indicators to flag suspicious claims. While these methods have been the backbone of fraud detection for decades, they come with significant drawbacks:
- Heavy reliance on historical data can result in overlooking emerging fraud patterns.
- Manual reviews are time-consuming and subject to human error.
- There’s a high cost associated with false alerts which can lead to customer dissatisfaction when legitimate claims are delayed.
According to the FBI, the insurance industry is inundated with over $1 trillion in premiums from more than 7,000 companies, making it a ripe target for fraud. This urgent scenario demands a method that balances predictive accuracy with operational efficiency, thus underscoring the necessity of machine learning techniques.
The Machine Learning Solution
1. Enhanced Predictive Analytics in Insurance
Machine learning excels in predictive analytics, allowing insurers to sift through vast datasets efficiently. These algorithms can analyze past claims to identify patterns and predict future fraudulent activity, increasing operational effectiveness.
Data from insurance claims can include various attributes such as categorical data (e.g., vehicle type, policyholder information), numerical metrics (e.g., claim amounts), and temporal data (e.g., incident dates). By utilizing predictive analytics in insurance, machine learning algorithms adaptively learn from incoming data, providing ongoing adjustments to fraud detection capabilities.
2. Real-Time Monitoring and Decision-Making
The real-time capabilities of machine learning mean that as soon as a claim is processed, AI can flag inconsistencies and alert the insurance team for further investigation. This dynamic response capability can significantly reduce potential losses.
By employing insurance fraud analytics frameworks, insurers can take immediate corrective actions without extensive delays. This includes utilizing AI-driven systems to monitor policyholder behaviors continuously and identify anomalies within their transaction data.
3. Feature Engineering and Selection for Optimal Model Performance
Machine learning models rely heavily on the quality of their input data. Through a rigorous process of feature engineering and selection, insurers can improve model accuracy significantly. This involves:
- Identifying relevant features that directly impact fraud probabilities.
- Iteratively refining the dataset to optimize model performance through techniques like PCA (Principal Component Analysis).
By enhancing the model’s capability to discern meaningful patterns, insurers strengthen their fraud detection processes, lowering the incidence of false positives and improving customer relations.
SimplAI Solutions: A Step Towards Smarter Fraud Detection
At SimplAI, we understand that the insurance industry requires innovative solutions to tackle the challenges of fraud detection. Our machine learning frameworks empower insurers to build complex, high-accuracy AI applications swiftly.
Conclusion: Embracing a Future Without Fraud
Detecting insurance fraud using machine learning is not just a technological upgrade; it’s a strategic necessity for modern insurers. By utilizing AI-driven analytics, insurance companies can efficiently combat fraud while maintaining customer trust and satisfaction.
As the battle against insurance fraud continues to evolve, the question remains: Is your organization ready to embrace machine learning in the fight against fraud?
To explore how SimplAI can help innovate your fraud detection strategies, visit us today!