Predictive Analytics for Customer Behavior in Banking
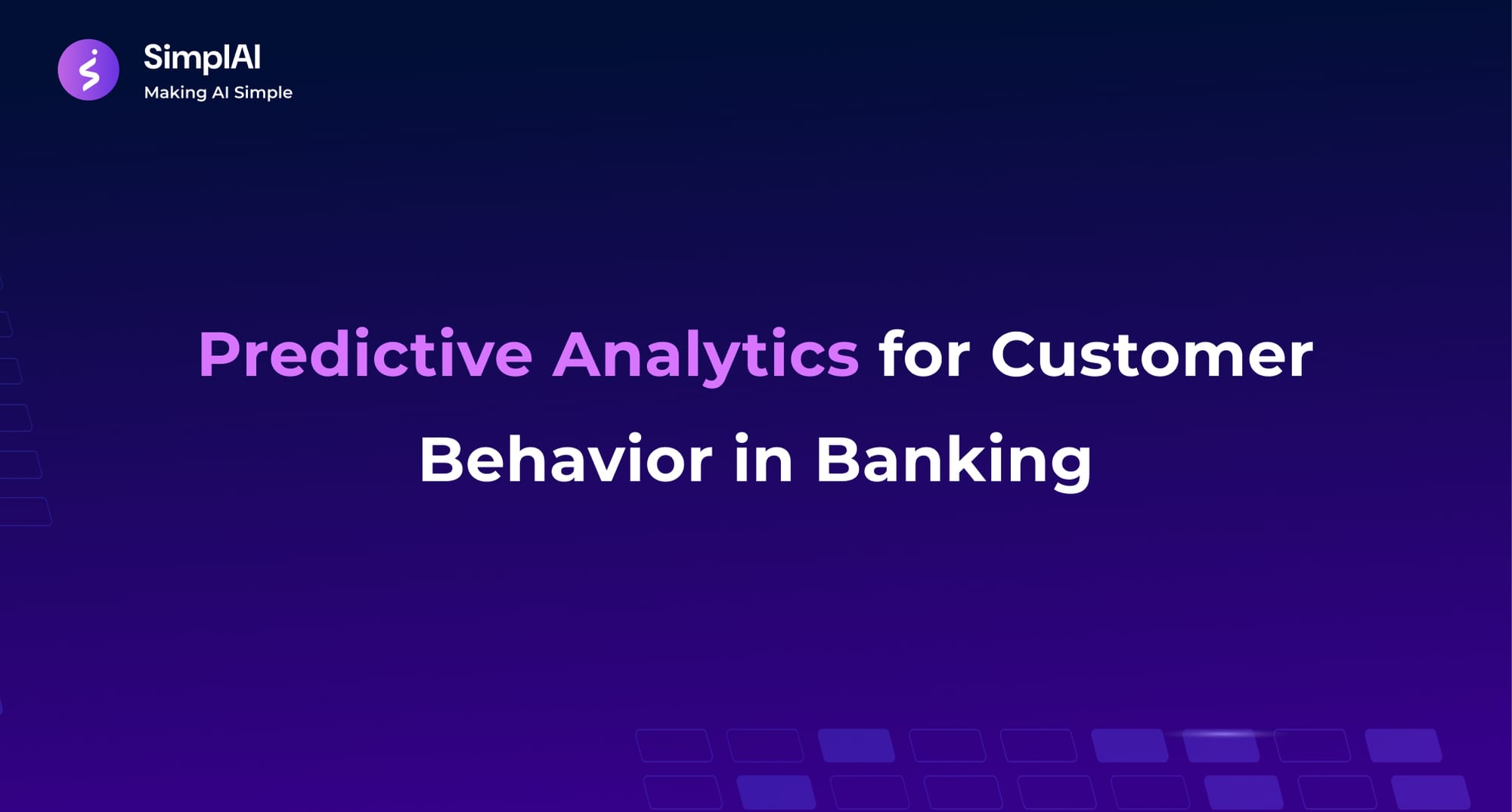
As we navigate through an increasingly competitive landscape, predictive analytics in banking has emerged as a game-changer, forging the path toward understanding and anticipating customer behavior. In an era where personalized banking experiences are not just preferred but expected, financial institutions are harnessing the power of customer behavior analysis AI to refine their offerings and enhance customer satisfaction.
Why Predictive Analytics Matters for Banking
The global predictive analytics market in banking is forecasted to grow markedly, with an estimated compound annual growth rate (CAGR) of 19.42% by 2030. This staggering growth is driven by the need for banks to combat rising threats such as fraud and to leverage large volumes of high-quality data for better decision-making. With predictive analytics, banks can analyze behavioral data more effectively, turning it into actionable insights that transform customer interactions.
Key Components of Predictive Analytics in Banking
Understanding how to implement and benefit from banking predictive modeling techniques is crucial. Here’s a look at the essential components:
1. Data Collection and Preparation
Data lays the groundwork for gaining reliable insights. Banks should focus on collecting high-quality data from diverse sources, ensuring accuracy and relevance.
2. Data Analysis and Modeling
Utilizing machine learning algorithms, banks can identify patterns in historical data. These predictive models allow institutions to foresee customer behaviors and potential risks.
3. Training and Evaluating Models
Continuous monitoring and evaluation of the predictive models ensure they remain effective and relevant. By fine-tuning these models, banks can enhance decision-making processes significantly.
4. Implementation of Predictive Models
Integrating predictive models into the bank's operational systems facilitates real-time decision-making. This integration is crucial for dynamic environments where customer needs may change rapidly.
5. Interpreting Insights
Translating complex data insights into practical solutions is vital. Banks must understand how to leverage predictions for marketing strategies and customer relations effectively.
Transforming Customer Insights with AI
Predictive analytics enables banks to gain deeper insights into customer preferences. By segmenting customers based on behavioral data, banks can tailor strategies that resonate with unique needs. This not only enhances customer engagement but also improves loyalty. For instance, banks can forecast potential churn rates and cultivate strategies to retain at-risk customers through personalized offers.
Use Cases of Predictive Analytics in Banking
Predictive analytics has a myriad of applications in the banking sector. These include:
- Customer Segmentation: Tailoring marketing campaigns to fit different customer segments increases engagement and satisfaction.
- Fraud Detection and Prevention: AI systems can analyze transaction patterns to identify suspicious activities promptly.
- Credit Evaluation: Advanced analytics enables banks to assess the creditworthiness of borrowers accurately, lowering the risk of defaults.
- Risk Management: Predictive tools allow institutions to anticipate potential risks, enabling proactive measures to protect assets.
- Churn Prediction: By analyzing past customer behavior, banks can implement measures to retain customers who are likely to switch to competitors.
SimplAI: Your Partner in Predictive Analytics
At SimplAI, we understand that implementing predictive analytics can be daunting.We specialize in creating complex, high-accuracy Agentic AI applications that empower financial institutions to leverage predictive analytics effectively. By aligning our solutions with your strategic objectives, we help banks unlock the full potential of their data, driving better customer experiences and operational efficiencies.
Conclusion
As predictive analytics continues to redefine the banking landscape, staying ahead of industry trends and customer expectations becomes more crucial than ever. By integrating customer segmentation AI tools and other advanced analytics capabilities, financial institutions can foster richer customer relationships and enhance their offerings.
What challenges do you foresee in implementing predictive analytics within your banking institution? Feel free to share your thoughts!
Ready to transform your banking services with predictive analytics? Explore SimplAI’s robust solutions and discover a faster, simpler way to build high-accuracy AI applications tailored for your needs.